In 1997, IBM’s supercomputer Deep Blue made history by defeating chess grandmaster Garry Kasparov, showcasing AI's ability to tackle highly complex problems. While this milestone highlighted AI’s potential for strategic decision-making, the technology has since evolved far beyond chess. Today, AI is transforming businesses of all kinds by automating and scaling decision-making processes that were once impossible for humans to manage on their own.
One of the most promising of these innovations is AI Decisioning, a new wave of AI technology designed to automate and optimize decision-making. Unlike generative AI, which creates text and visuals, AI Decisioning empowers businesses to utilize AI to make intelligent decisions at a beyond-human scale. It’s the same technology that powers many of the hyper-personalized experiences we interact with daily from big tech companies, continuously learning and improving with each customer interaction.
So, what exactly is AI Decisioning? How does it work, and why does it matter for modern marketers? This blog unpacks everything you need to know about AI Decisioning, including its evolution, importance, and how marketers can use its power to drive their business and marketing efforts forward.
What is AI Decisioning?
AI Decisioning refers to the use of advanced machine learning—specifically reinforcement learning and AI agents—to make intelligent, data-driven decisions.
These systems continuously test, learn, and optimize actions to achieve specific business goals, such as driving customer engagement, increasing revenue, or improving lifetime value (LTV). Unlike traditional A/B testing or manual rule-based systems, AI Decisioning operates on a continuous experimentation loop, delivering insights and optimization at a scale that would be impossible for humans to achieve manually.
Think about how today’s leading tech companies like Google, TikTok, and Amazon have redefined customer experiences through hyper-personalization:
- Google Ads: Determines the most relevant ad for each user, optimizing for clicks and conversions to maximize advertiser ROI.
- TikTok: Curates feeds tailored to individual interests, keeping users engaged longer and driving platform loyalty.
- Amazon: Powers product recommendations, predicting items you’re most likely to purchase, enhancing customer satisfaction, and boosting repeat purchase revenue.
For marketers, AI Decisioning platforms offer a new way to engage with their millions of customers on a 1:1 basis. Instead of sending the same email blast to everyone on a list or running basic A/B tests, AI learns from each customer interaction and curates highly personalized experiences based on each user’s behaviors and preferences.
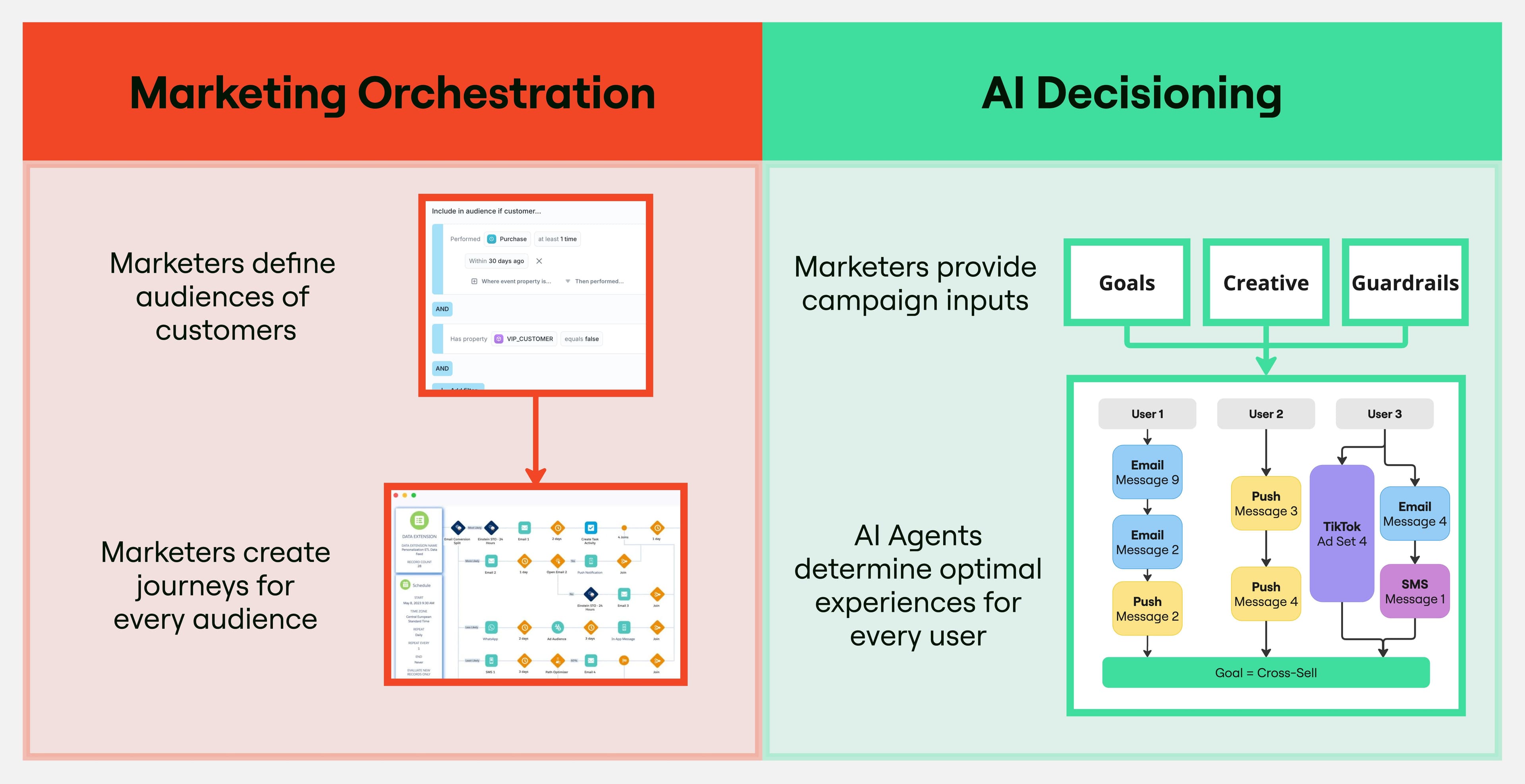
The rise in AI Decisioning systems
AI Decisioning is the result of years of progress in artificial intelligence, but it’s much more than a tech buzzword. While generative AI often grabs headlines for creating lifelike images and videos, AI’s ability to make smarter decisions is the real game-changer. Big tech companies have been using AI behind the scenes for years to solve complex problems and create experiences that keep customers coming back.
Here’s how some of the most innovative companies in the world use decisioning to power their businesses:
- Uber: Handles over 10 million decisions per second to manage ride ETAs, match riders with drivers, rank UberEats feeds, and detect fraud. These decisions ensure smooth, reliable experiences for users.
- Duolingo: Keeps users coming back with personalized push notifications. Its system decides which message will resonate most with each user, boosting app engagement and encouraging free users to upgrade to premium.
- Netflix: Recommends content tailored to individual tastes. By deciding which shows, movies, or games to feature, Netflix keeps users watching and coming back for more.
For years, only companies with huge budgets and massive engineering teams could build decisioning systems like these. They required complex infrastructure, specialized expertise, and access to enormous amounts of data, so this technology was mostly limited to big tech.
Today, things are different. Thanks to cloud data platforms like Snowflake, Databricks, and Google BigQuery, businesses of all sizes can finally harness the power of AI Decisioning. These platforms make it easier than ever to organize and access data, while AI Decisioning platforms like Hightouch give marketers the decisioning capabilities they need to create more personalized experiences for their customers.
AI Decisioning combines the latest advancements in data and machine learning to help businesses move beyond one-size-fits-all marketing. Now, marketers can use decisioning to automate complex processes and deliver personalized experiences at scale—just like big tech companies.
How does AI Decisioning work?
AI Decisioning is powered by three primary technologies: AI agents, reinforcement learning (RL), and large language models (LLMs). Together, these enable businesses to automate complex decisions and deliver continuously improving, personalized experiences at scale.
AI Agents
At the core of AI Decisioning are AI agents—autonomous programs designed to analyze data, make decisions, and learn from the results. Each agent focuses on specific tasks, such as recommending the best product, determining the optimal time and channel for a message, or personalizing an offer. These agents act as "digital marketers" for each of your customers, working tirelessly behind the scenes to test and refine strategies and make decisions faster and more accurately than any human could. For example, an AI agent might decide to send an email with a 15% discount to one customer while pushing a personalized SMS to another based on their behavior.
Reinforcement Learning
While AI agents handle the execution of decisions, reinforcement learning ensures those decisions get smarter over time. Reinforcement learning works by continuously analyzing the outcomes of actions and identifying patterns that lead to success or failure. For instance, if offering a discount via email results in higher engagement, reinforcement learning learns from this success and allows the AI agents to adjust future decisions accordingly. Unlike static rules or basic A/B tests, reinforcement learning creates a continuous feedback loop where every decision contributes to the system’s improvement, ensuring that actions become more effective with each interaction.
Large Language Models
Large language models (LLMs) add a layer of contextual understanding that takes AI Decisioning to the next level. LLMs autonomously analyze and tag content—like the tone, structure, and style of an email or push message—to make sure it resonates with each customer. For instance, an LLM might tag a piece of content so that the AI agents know to use a playful tone for younger audiences while recommending a more formal style for professionals. This added intelligence allows the system to learn from a vast amount of data, delivering the most relevant and effective experiences for every individual.
Bringing it all together: An example
Imagine an online pet retailer using AI Decisioning to increase repeat purchases:
- The marketing team selects the desired outcome and inputs their treatment components, including which channels they want to communicate with users on, message templates, product catalogs, and copy variants.
- AI agents analyze customer data to understand each shopper's preferences. For a customer who recently purchased dog food, an agent determines that a promotional offer for dog treats might be most appealing.
- Reinforcement learning ensures the system improves over time. If previous campaigns showed that customers respond better to offers sent in the afternoon, the reinforcement learning algorithm adjusts the timing of the promotional message to optimize engagement.
- Large language models refine the model by providing the context of each message. The LLM ensures that the tone of the email aligns with the customer’s profile—for example, a friendly and enthusiastic tone for young pet lovers.
Why is AI Decisioning so important?
Traditional marketing often treats people as groups rather than individuals. Ads, emails, and landing pages are designed and optimized for broad audience segments. This “good for most” approach rarely delivers optimal results because it fails to address the unique needs and preferences of individual customers.
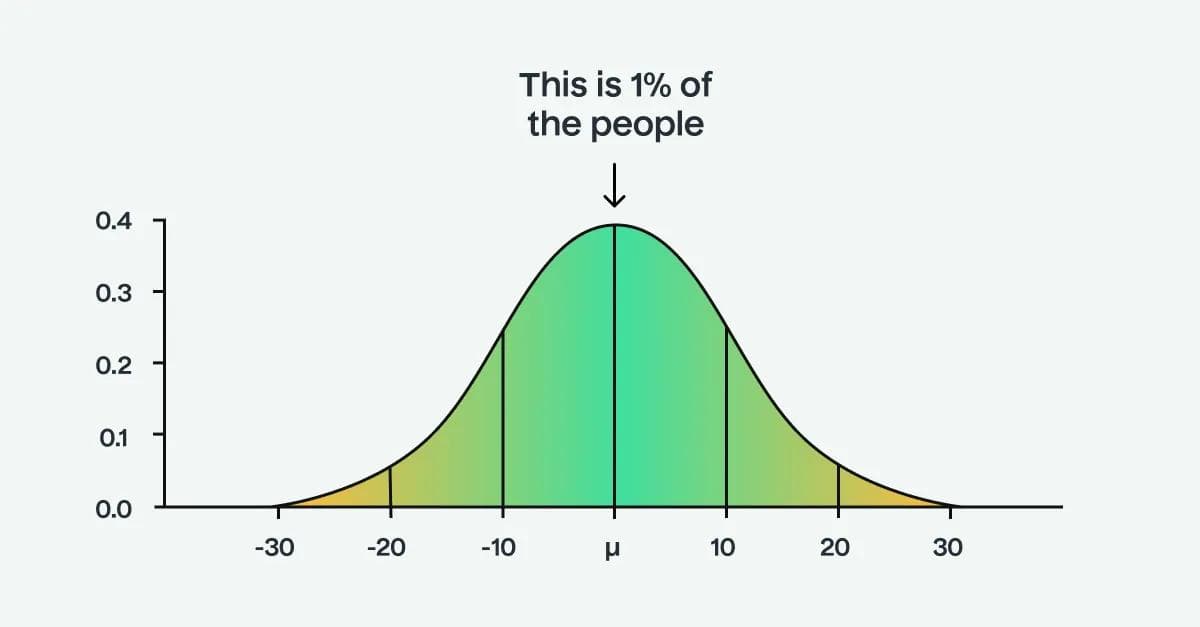
AI Decisioning changes this. By leveraging the power of data and AI, businesses can shift from strategies that are "good for most" to "calibrated for each." This mirrors what we’ve already seen in other industries:
- Newspapers have evolved into personalized newsfeeds.
- Linear TV has been replaced by on-demand streaming platforms.
- Traditional dating has given way to matchmaking apps that tailor experiences to individual preferences.
The same transformation is happening in marketing. According to McKinsey, companies that personalize their marketing efforts can drive 40% more revenue than those that don’t.
AI Decisioning makes this level of personalization possible at scale, allowing businesses to engage with customers in previously unattainable ways.
Use cases for AI Decisioning
AI Decisioning can be applied to any situation with clear inputs and outputs. Outputs are business goals like increasing the number of users who make a second purchase or increasing the conversion rate of a website page. Inputs include the components that go into a user experience treatment, like message templates, product catalogs, and copy variants.
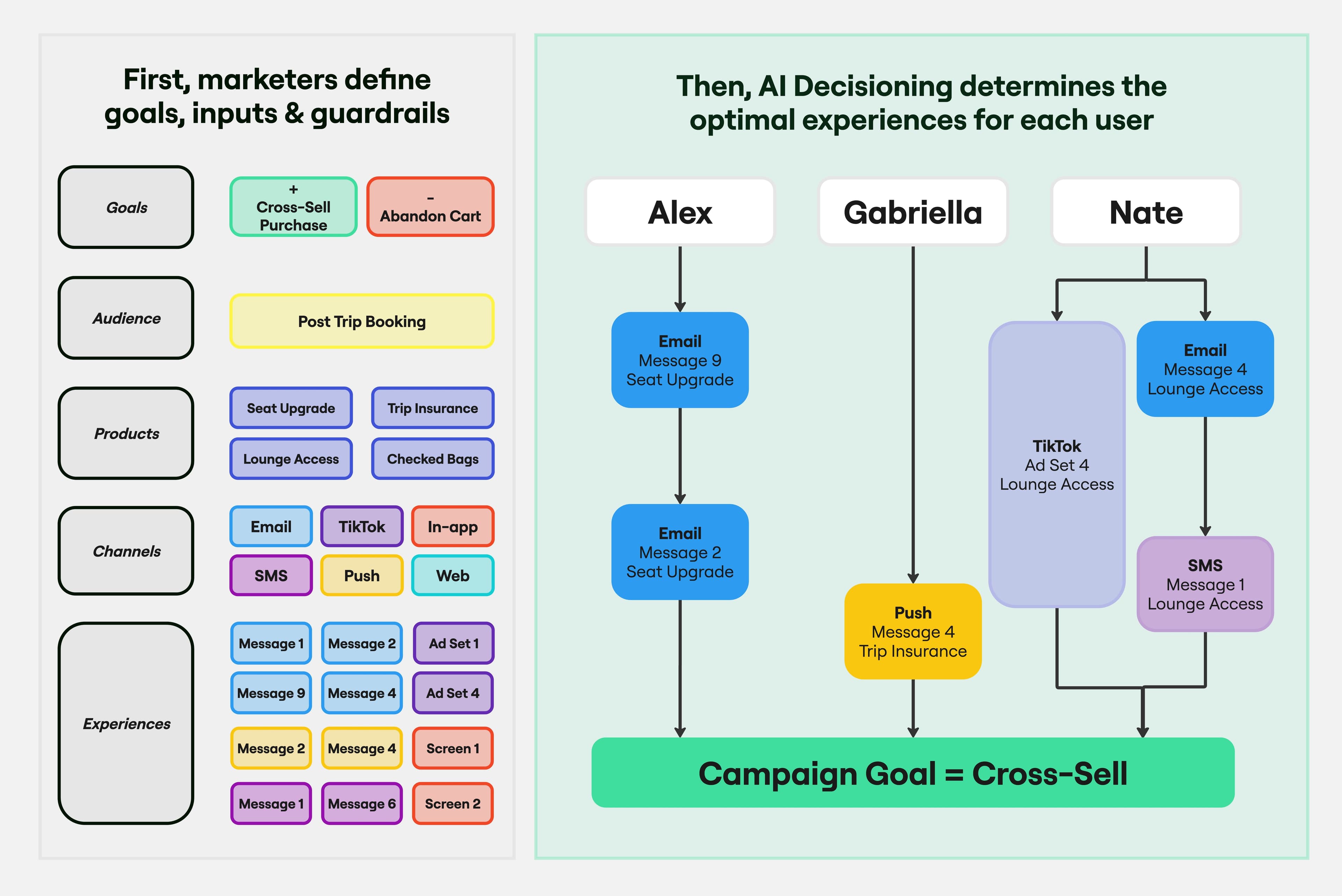
With AI Decisioning, every customer journey is dynamically personalized, ensuring more relevant interactions and optimized cross-sell opportunities.
Here are two key areas where AI Decisioning is making an impact for teams today:
Lifecycle Marketing
Marketers use email, SMS, and push notifications to guide customers through their journey, whether it’s driving a second purchase, cross-selling a related product, or winning back disengaged users. With AI Decisioning, these campaigns become smarter and more effective. For example:
- A pet retailer can tailor grooming service offers based on the breed of a customer’s dog.
- An e-commerce store can recommend products based on past purchases, personalizing discounts, and messaging for each user.
- A streaming service can use AI Decisioning to re-engage inactive users with curated recommendations that match their viewing history.
A few practical examples of how these use cases look in practice:
Use Case | Traditional Approach | AI Decisioning: Sam | AI Decisioning: Alex | Outcome |
---|---|---|---|---|
A sports clothes e-commerce store wants to drive a customer to make a second purchase | All users receive an email two hours after an order with a 10% discount to add a best-selling to their order | Sam receives an email a day later offering a discount on a pair of sneakers that he doesn’t own | Alex receives an SMS, followed by an email two days later for gaming-themed clothing | Increased customer lifetime value (LTV) |
A pet retailer wants to cross-sell more products | All users get an offer for in-store grooming for their dog | Sam receives an email for a bath, brush, and blowdry aimed at French bulldogs | Alex receives a direct mail promotion for a full groom aimed at poodles | Increased customer LTV and recouped customer acquisition cost (CAC) |
A streaming company wants to win back disengaged users | All users receive an email highlighting new shows or movies | Sam is sent a 3-email win-back sequence, starting on a Tuesday and ending on a Saturday, with the last email offering a free month subscription | Alex gets targeting on Facebook, showcasing the new release of fitness, cooking shows, and gaming content based on his peak engagement time | Reduce churn |
Onsite & In-App Experience Optimization
AI Decisioning enhances user experiences by customizing app interfaces, website pages, and content recommendations. For example:
- An airline can show frequent travelers personalized upgrade offers.
- A food delivery app can highlight deals from a customer’s favorite restaurants during peak ordering times.
A few practical examples of how these use cases look in practice:
Use Case | Traditional Approach | AI Decisioning: Gabs | AI Decisioning: Taylor | Outcome |
---|---|---|---|---|
An airline wants to increase seat upgrade sales | All users see a 10% off banner on the website for seat upgrades | Gabs sees a personalized banner offering a 15% discount on seat upgrades due to his frequent travel history | Taylor sees the banner partway through the checkout process, with an offer focusing on budget-friendly options | Increased customer LTV |
A food delivery app wants more users to order via the app | All users receive an in-app notification to make an order | Gabs receives an in-app notification that his favorite gourmet restaurant has a new menu available | Taylor gets an in-app notification at lunchtime about an affordable meal deal from an eco-friendly restaurant that includes a student discount | Increased user engagement |
Best AI Decisioning tools
Previously, AI Decisioning was only accessible to big tech companies with the resources to build in-house solutions. Today, several platforms make this technology available to businesses of all sizes:
- Hightouch is one of the few AI Decisioning solutions built to securely use all of your existing data and marketing tools. The platform plugs into your existing tech stack, learns from all your data, and lets you autonomously run experiments to deliver the best possible user experiences across 300+ native integrations.
- Offerfit is another Martech tool that integrates with your existing tech stack. It provides AI capabilities to personalize content for each individual using your first-party data by generating content variants for different mediums and understanding what the best message, product, incentive, timing, and frequency is for each customer.
- Moveable Ink helps you use AI to curate email content tailored to individual customer interests to produce individualized experiences. It allows for optimizing multiple business objectives by determining optimal time, frequency, and content for each user.
- Aampe is an agentic infrastructure that automates your CRM to send the best message to each of your customers across channels such as push notifications, SMS, and email. It requires a CPaaS connection so you can send messages and a CDP connection to track impact and has limited integrations.
Final thoughts
The era of one-size-fits-all marketing is over. With AI Decisioning, businesses can deliver truly personalized experiences at scale, replacing outdated methods like A/B testing with always-on optimization. Whether your goal is to drive customer engagement, increase lifetime value, or improve operational efficiency, AI Decisioning provides the tools to succeed.
If you’re ready to transform your marketing with AI Decisioning, book a demo with Hightouch.