Data teams need to break out of their bubble
Data teams and stakeholders are often deeply siloed from each other, and the data team/marketing team divide is a powerful example of this dysfunction.
Mary MacCarthy
November 17, 2022
|9 minutes

I find many of the discussions I’m hearing and reading in the modern data stack community to be too inward-facing. And it’s a perspective which, I fear, reflects how data teams or data practitioners often function within companies–with a detachment from business outcomes.
The discussions I’m referring to are the bold statements on Linkedin about data quality and observability, the "nth" Twitter discussion of what a semantic layer is and why we need one, and the endless chatter about how data-products-data-governance-data-mesh is the future–as heard echoed in yet-another-Slack-community, or at yet-another-data-conference.
I’m not questioning the technical truth or intellectual authenticity of any of these debates, most of which are led by brilliant minds. I just feel that so much of the subject matter doesn’t tie closely enough to the entire reason for having a data team–which is to drive business value.
There’s a point at which the modern data stack “thought leadership” and hot conversations start to feel like walking down a hall of mirrors. After a while, you’re not seeing anything new. And it starts to feel a bit like–navel-gazing. Especially in this current moment when market, economic, and business pressures are all too real.
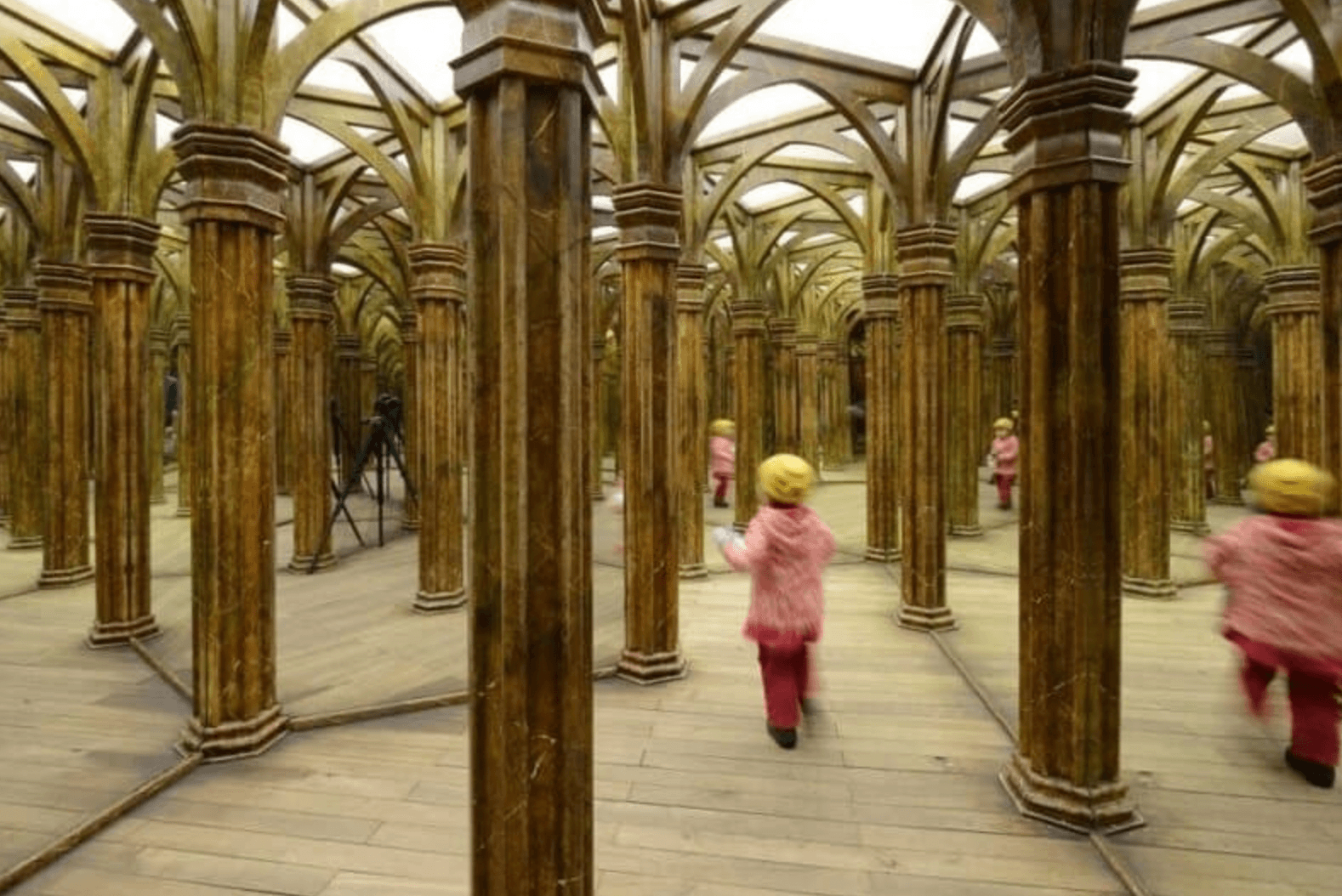
Navel Gazing
We’re all keenly aware of the massive lay-offs happening around us. We know that deep cost-cutting is likely on the horizon, and that businesses everywhere are trying to do more with less. It’s a time in which EVERY team should be laser-focused on just one thing: adding value to the business.
I think it’s time for data teams to break out of their bubble and get a bit more business-savvy.
In this economy–our jobs might depend on it.
Forget Data Silos, The Bigger Problem is Siloed Data Teams
Most data practitioners are incredibly busy, working in fast-paced roles–doing the architecting and analyzing of the data for multiple stakeholders, usually in response to incessant requests from business teams.
It’s not their fault that they’re often deeply siloed from the stakeholders they’re serving; that’s simply an operational reality in many organizations.
Let’s explore just one example of the gap between data teams and other stakeholders: data teams and marketing teams.
I’ve spoken to dozens of people who work in data and marketing, across companies of all sizes. Nearly all of them describe a deep divide between their marketing and data team.
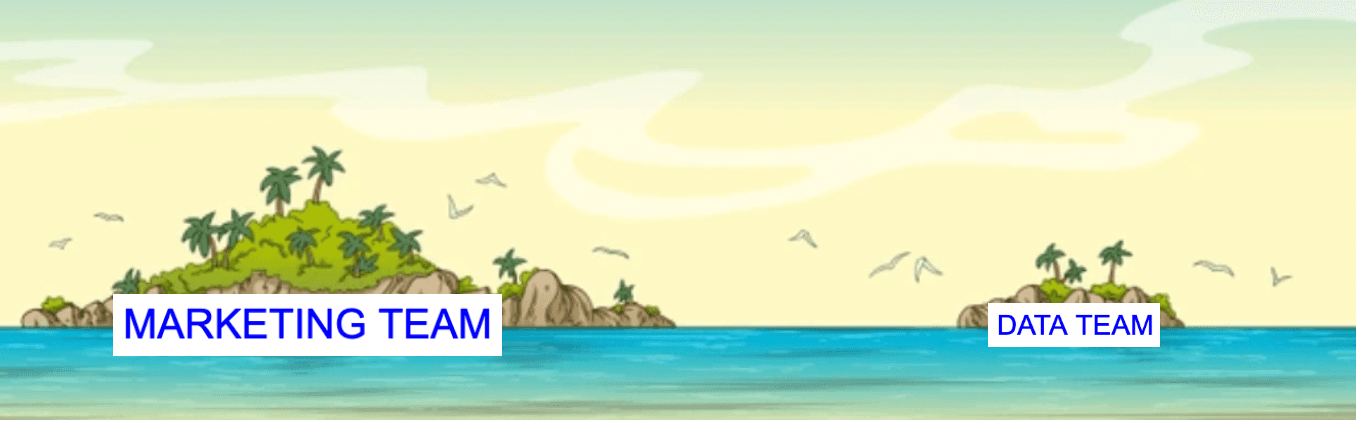
The Great Divide Between Data and Marketing Teams
Data consultant Timo Dechau says that siloes between marketing teams and data teams are the norm, not the exception:
The major problem is that marketing and data each have distinct objectives. Marketing’s mission is to run campaigns and experiments, to generate pipeline and ultimately revenue. All they really care about from the data team is: can they do what I need in a timely manner? If the data team tells them a project will take six sprints, their reflex is often to hire an agency or freelancers rather than to wait on the data team.
Do marketing’s demands further the data team’s objective of building a solid foundation for their modern data stack–with all stakeholders working from a single source of truth stored in a cloud data warehouse? Not at all! But why should the marketing team care? If their current way of working leads to demonstrable results for their campaigns, why should they alter it?
I would add to this: oftentimes, marketing teams don’t even need to source anyone outside their department in order to build a technical solution. They often have embedded analysts who are extremely technically savvy–people who can come up with “in-house,” ad-hoc solutions for much of their team’s needs.
Brian Pacini, FP&A Business Intelligence Director at RingCentral, says this is a reality he’s seen in multiple companies:
An embedded analyst is usually facing pressure from business VPs who want stuff done fast and who don’t care if it’s done thoroughly. So, when you have marketing pulling their own data in this way, they’re going to make mistakes. It’s unlikely they’ll build a system that can be productionalized, or with a high level of data quality.
Sure, marketing is motivated more by short-term wins than by concern for working with data and data infrastructure that’s foundationally strong. But again–who can blame them? If they’re able to answer the questions their VPs are asking and show that their campaigns are leading to revenue, then why change?
If you stop and think about it, convincing a marketing team to work more closely with a data team is a really big ask. It’s kind of natural that the teams don’t easily align: not only do they not have shared goals, but they also don’t work at the same pace. Marketing has to move fast and show results. Data teams, in contrast, have to prioritize the design and building of foundational workflows and infrastructure that scale (and don’t leave a trail of technical debt in their wake 😅).
Example of Currenty Absurdity: Modern Data Stack vs. The Martech Stack
What really got me thinking about the divide between marketing and data teams is the discourse around just one tool–something called a “CDP.”
“CDP” stands for “customer data platform.” It sounds like a tool that’s part of the modern data stack. But, weirdly, most data practitioners I’ve spoken to don’t know what a CDP is.
Despite having “data” in its name, a CDP is actually one of the many tools in marketing’s own “martech” stack.
Generally, it’s a very expensive tool: companies shell out hundreds of thousands of dollars for multi-year contracts with CDP providers.
The exact definition of a CDP can vary, but here are the basics: a CDP is a database that stores all the information you have about your customers, mostly collected via front-end tracking on your website and/or in your product. The CDP also includes a software interface with highly valuable functionalities for marketers. These can include:
- Doing identity resolution (determining if User A on their website is actually the same person as User B on their website)
- Creating segments of users–groups that can be synced from the CDP to various marketing and advertising platforms, to run targeted campaigns.
I know, you’re probably thinking what I’m thinking: why is a CDP part of the martech stack and not the data stack?
After all, storing customer data, and sophisticated data processing like identity resolution and segmentation, is data work. And even if CDPs enable some of the work in a non-technical, no-code way–surely there should be some oversight from the data team? Or at least a close collaboration between marketing and data?
I’m not trying to argue for or against CDPs (my colleagues spend plenty of time discussing the future of CDPs and where to manage things like Identity Resolution.) My argument is that once an organization has reached the size at which a marketing team seeks out a tool like a CDP, it becomes problematic if the marketing team and the data team are not working hand-in-hand.
Given how advanced the data capabilities are of CDPs and similar marketing platforms, it’s absurd to be talking about a data stack and a separate martech stack. After all, these tech stacks are simply a means to an end: delivering a better customer experience and ultimately driving revenue.
All the decisions around a tool or platform–from the purchase and implementation, to how it can be used in an optimal way, to how it fits with other underlying architecture and software–should not be marketing-led. Rather, the evaluation should happen at a business level, and be jointly handled by marketing and data.
It sounds obvious, yet that’s not what happens in most companies.
In some organizations, the data team is not even aware of which CDP the marketing team uses. Which is, frankly, insane. It means that huge amounts of time and money are being invested into marketing’s own data “shadow infrastructure,” without insight from the people whom the company is paying as data professionals!
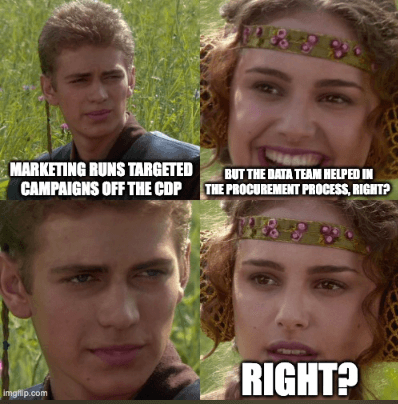
Shadow Infrastructure
What’s more, CDPs often end up requiring significant onboarding and maintenance work by members of the data team. In the words of Kelly Burdine, Director of Data Science and Analytics at Wellthy:
A data team can end up forced to work on a platform for which they were not consulted in the decision to purchase–and which, had they been consulted, they may very well have advised against.
First Step in Breaking Out of the Data Bubble: Learn to Speak #Martech
I started this article by suggesting that data teams need to break out of their bubble and become more business-savvy. I think a great way to start is this: attempt to bridge the divide with just one of your stakeholders–the marketing team.
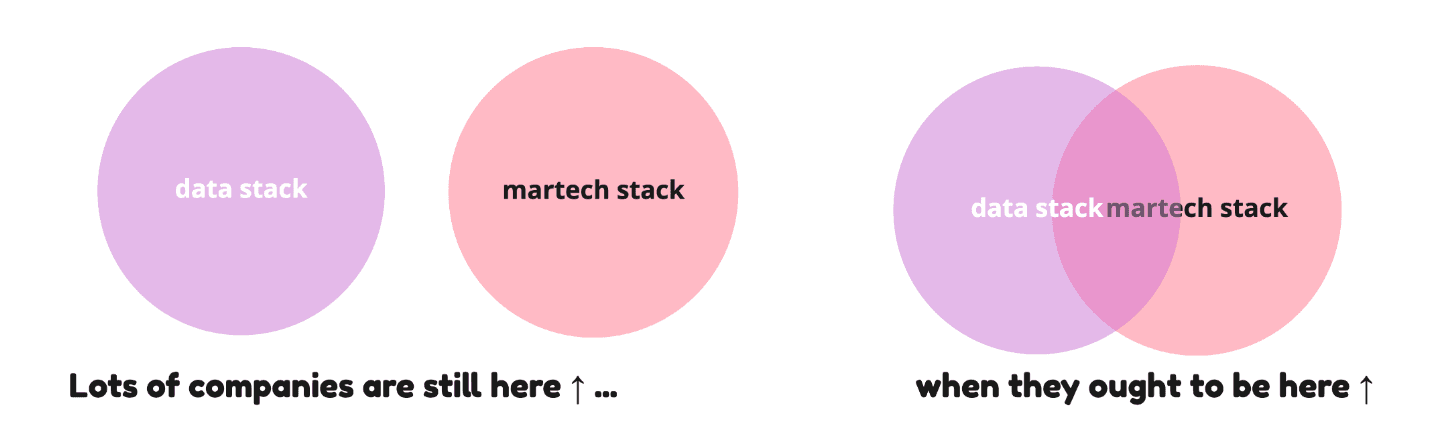
Combining Data and Marketing
Step away from the modern data stack for a bit, and learn more about the martech stack and the martech lexicon. Here’s a checklist of questions you can start with:
- How does the marketing team measure success? What are the gaps here?
- What tools does your marketing team use? How do they use them?
- What data does the marketing team already have access to? Is it complete? Is it accurate?
- What other stakeholders does marketing interact with for its data needs, and what does that interaction look like?
- Being aspirational, what would the marketing team ideally want access to?
- What would this enable?
If the data team doesn't already know the answer to these questions, there's potentially a lot of opportunity and business value being left on the table.
At a minimum, you’ll learn how to better serve your marketing team requests.
But more likely, you’ll be empowered to push for deeper, transformative collaboration–work that helps marketing to achieve greater results thereby demonstrating the business value of the data team.
That way, you can say more than “I maintained those pipelines” or “built those dashboards.”
You can also take credit for business outcomes, like “our data modeling led to more accurate customer segmentation, which boosted the success of marketing’s campaigns by ‘x’ percent.” Or, “our self-serve data infrastructure has saved the marketing team ‘x’ number of hours per week, and has also ensured that their data is updated every half hour rather than twice a week.”
THOSE are statements of a data practitioner who has burst out of the data bubble and learned to think in terms of business outcomes. And it’s what we all should be striving for.